Challenges in Generalizing AI Models for Mental Health: A Perspective for Psychiatry Tech
Artificial Intelligence (AI) has the potential to revolutionize healthcare, including the field of psychiatry. However, recent research suggests that AI models for mental health pose significant challenges. In particular, the lack of generalizability of these models is a major concern.
What Is Generalizability?
Generalizability refers to the ability of an AI model to perform accurately on data that it has not seen before. In other words, a generalizable model should be able to recognize patterns in new datasets that it has not previously encountered.
Generalizability is essential for AI models to be effective in clinical settings. Without generalizability, AI models may perform well on the data used to train them, but could fail to make accurate predictions in real-world situations. This could have serious consequences for patients.
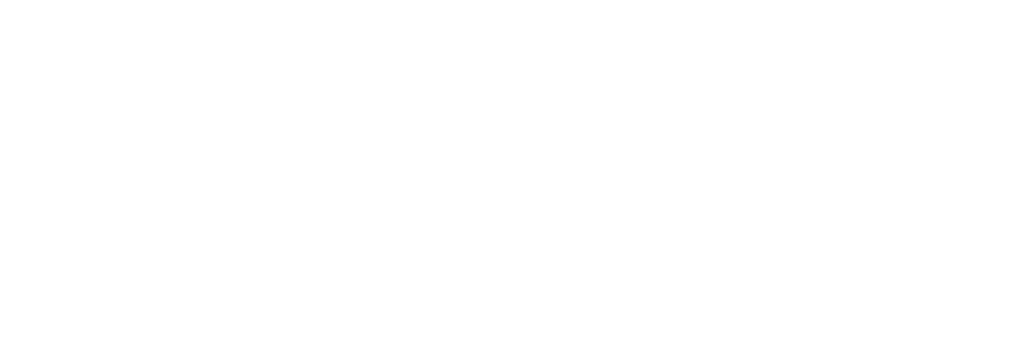
Discover The World's MOST COMPREHENSIVE Mental Health Assessment Platform
Efficiently assess your patients for 80+ possible conditions with a single dynamic, intuitive mental health assessment. As low as $12 per patient per year.
The Challenges of Generalizing AI Models in Mental Health
One of the challenges of generalizing AI models in mental health is the lack of diversity in training data. Mental health datasets tend to be relatively small and biased towards specific populations, such as college students or patients with particular diagnoses. This can lead to AI models that are only accurate in predicting outcomes for these specific groups, but unable to generalize to other populations.
Another challenge is the complexity of mental health data. Mental health data is often messy and difficult to understand, with many different factors contributing to outcomes. AI models may struggle to identify important patterns in data, particularly when those patterns are not easily visible to human clinicians.
Finally, there is the challenge of explaining the outcomes of AI models to clinicians and patients. AI models use complex algorithms to make predictions, which can be difficult to understand for people without a background in statistics or computer science. Clinicians and patients need to be able to trust AI models and understand how they work in order for them to be used effectively in clinical settings.
What Can Be Done to Improve Generalizability?
Improving generalizability in AI models for mental health requires a multi-disciplinary approach. This includes:
- Collecting larger, more diverse datasets that better reflect the populations of interest.
- Working with clinicians and patients to identify important factors in mental health outcomes and incorporating this knowledge into AI models.
- Developing methods for explaining the outcomes of AI models in ways that are understandable to clinicians and patients.
In addition, researchers need to continue developing new algorithms and techniques that can better handle complex mental health data. This includes developing models that can integrate different types of data (such as clinical data, genetic data, and imaging data) to improve predictions.
Conclusion
AI models have the potential to transform mental health care, but their lack of generalizability is a significant hurdle to overcome. By working together, researchers, clinicians, and patients can develop AI models that are more accurate, effective, and trusted.
What are your thoughts on the challenges of generalizing AI models for mental health? Share this post and join the conversation on Psychiatry Tech!